Advisor: Yehonadav Bekenstein
Contact: bekenstein@technion.ac.il
Project description:
Halide perovskite quantum dots (e.g. CsPbBr3) are a promising class of photoactive materials with potential applications as quantum emitters, spintronic devices, sensors, as well as in photovoltaics, lighting, display technology, photocatalysis, etc. The discovery of new materials of this group fitted for these applications requires optimizing composition, synthesis conditions, and post-processing. It sums up into a multi-parameter space, where a direct trial-and-error approach would require thousands of experiments. We apply machine learning-based experiment planning combined with robotic synthesis to address this challenge and develop a framework for fast and efficient halide perovskites discovery.
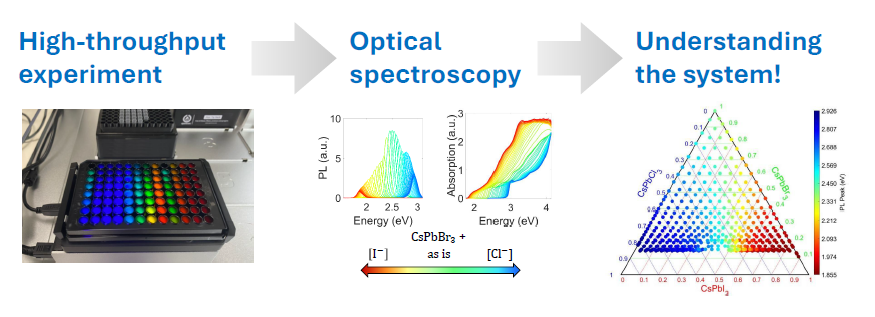
We invite you to contribute to the future of quantum materials by:
- Mastering advanced optical and structural materials characterization techniques
- Inventing the automation for the data flow produced by the high-throughput experimentation
- Implementing machine-learning decision making
- Improving our setup for automated synthesis, implementing advanced robotic features
- Adopting workbench chemistry and making it suitable for a robot
- Treating the robot well so it won’t rise against us